Machine Learning in Demand Forecasting: Revolutionizing Predictive Analytics
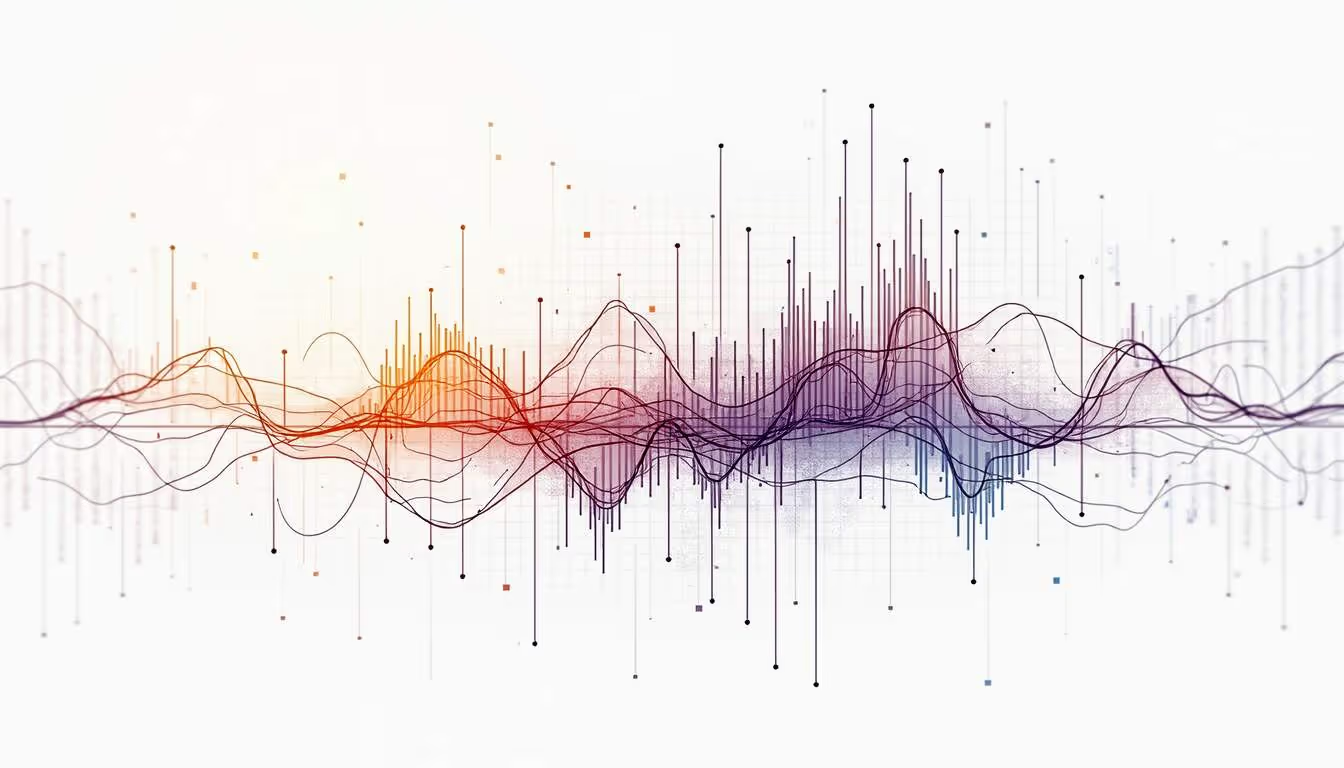
In today's fast-paced business environment, accurately predicting consumer demand is more critical than ever. Companies are turning to advanced technologies to gain a competitive edge, and machine learning in demand forecasting is at the forefront of this transformation. By leveraging sophisticated algorithms and data analytics, businesses can enhance their forecasting accuracy, optimize inventory management, and ultimately improve their bottom line.
Understanding Demand Forecasting
Demand forecasting is the process of predicting future consumer demand for a product or service. It involves analyzing historical data and market trends to make informed decisions about production, inventory, and supply chain management. Accurate demand forecasting is crucial for businesses to minimize costs, reduce waste, and meet customer expectations.
Traditional demand forecasting methods often rely on statistical models and human intuition. However, these approaches can be limited by their inability to process large volumes of data and adapt to rapidly changing market conditions. This is where machine learning comes into play, offering a more dynamic and data-driven approach to forecasting.
The Role of Machine Learning in Demand Forecasting
Machine learning algorithms excel at identifying patterns and relationships within complex datasets. By analyzing vast amounts of data from various sources, such as sales records, market trends, and consumer behavior, machine learning models can generate more accurate and timely demand forecasts. This enables businesses to respond more effectively to market fluctuations and customer needs.
One of the key advantages of machine learning in demand forecasting is its ability to continuously learn and improve over time. As new data becomes available, machine learning models can update their predictions, ensuring that forecasts remain relevant and accurate. This adaptability is particularly valuable in industries with volatile demand patterns, such as fashion and technology.
Benefits of Machine Learning in Demand Forecasting
Improved Forecast Accuracy
Machine learning models can process vast amounts of data and identify complex patterns that traditional methods may overlook. This results in more accurate demand forecasts, allowing businesses to make better-informed decisions about production, inventory, and distribution. Improved forecast accuracy can lead to reduced stockouts, minimized excess inventory, and enhanced customer satisfaction.
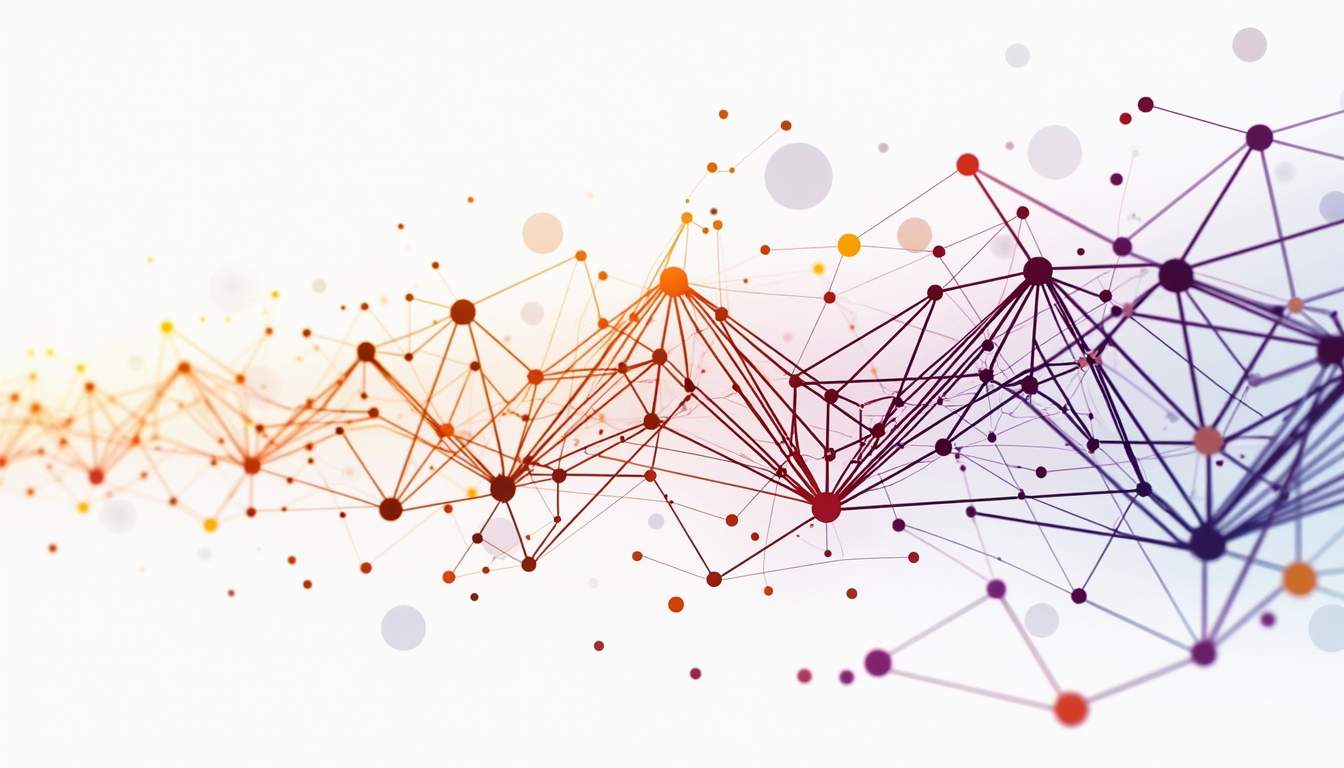
Enhanced Inventory Management
Accurate demand forecasts enable businesses to optimize their inventory levels, ensuring that they have the right products in the right quantities at the right time. This reduces the risk of overstocking or understocking, which can lead to increased costs and lost sales. Machine learning can also help identify slow-moving or obsolete inventory, allowing companies to make data-driven decisions about markdowns and promotions.
Cost Reduction and Efficiency
By improving forecast accuracy and optimizing inventory management, machine learning can help businesses reduce costs and increase operational efficiency. This includes lowering carrying costs, minimizing waste, and reducing the need for expedited shipping. Additionally, machine learning can streamline supply chain processes, leading to faster response times and improved resource allocation.
Implementing Machine Learning in Demand Forecasting
Data Collection and Preparation
The first step in implementing machine learning for demand forecasting is to gather and prepare relevant data. This includes historical sales data, market trends, and external factors such as economic indicators and weather patterns. Data quality is crucial, as inaccurate or incomplete data can lead to unreliable forecasts. Businesses should invest in robust data collection and cleaning processes to ensure the integrity of their datasets.
Choosing the Right Machine Learning Model
There are various machine learning models available for demand forecasting, each with its strengths and weaknesses. Common models include linear regression, decision trees, and neural networks. The choice of model depends on factors such as the complexity of the data, the desired level of accuracy, and the computational resources available. Businesses should experiment with different models and fine-tune their parameters to achieve the best results.
Integrating Machine Learning into Business Processes
Once a suitable machine learning model has been developed, it should be integrated into existing business processes. This involves aligning the model's outputs with decision-making workflows and ensuring that relevant stakeholders have access to the forecasts. Businesses should also establish feedback mechanisms to continuously monitor and improve the model's performance.
Challenges and Considerations
Data Privacy and Security
As machine learning models rely on large volumes of data, businesses must ensure that they comply with data privacy and security regulations. This includes implementing measures to protect sensitive information and obtaining the necessary consents from data subjects. Failure to address these issues can result in legal and reputational risks.
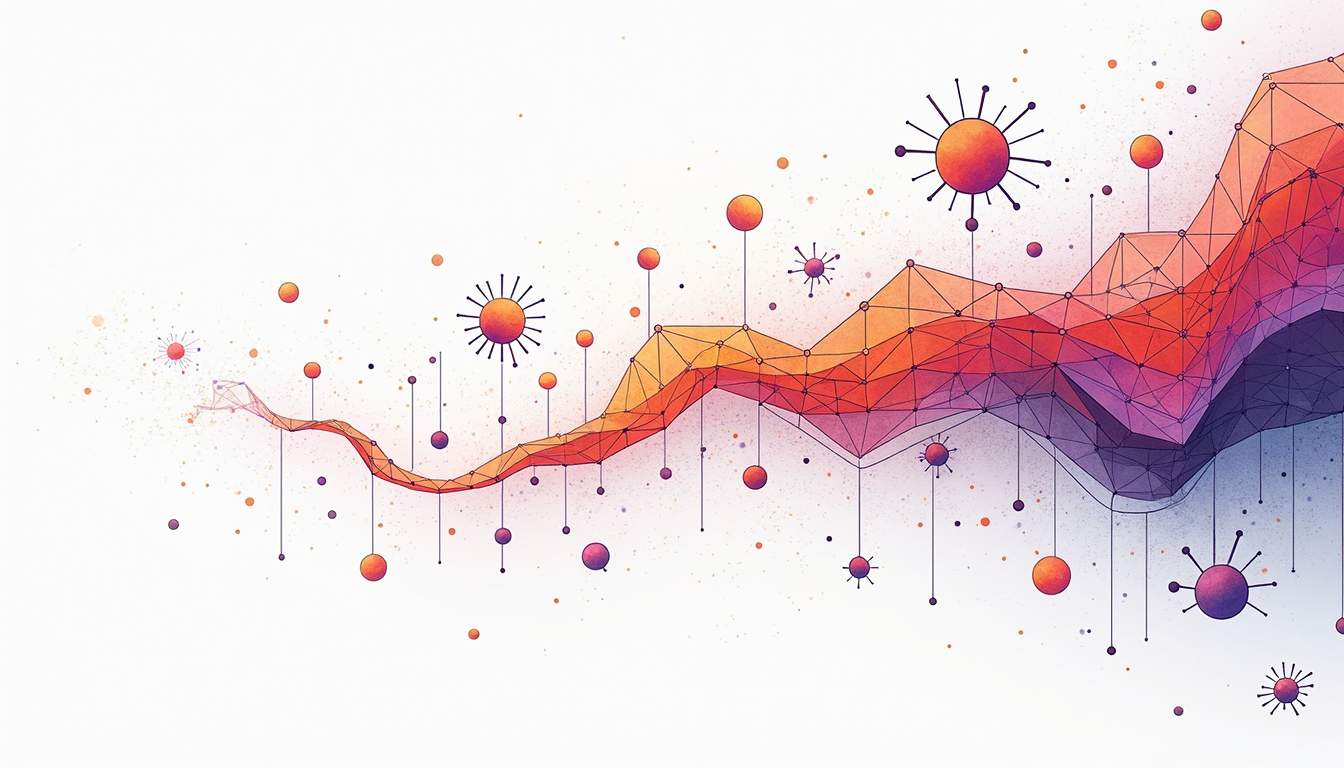
Scalability and Infrastructure
Implementing machine learning in demand forecasting requires significant computational resources and infrastructure. Businesses must invest in scalable solutions that can handle large datasets and complex calculations. Cloud-based platforms offer a flexible and cost-effective option for scaling machine learning capabilities.
Change Management and Adoption
Introducing machine learning into demand forecasting processes may require a cultural shift within the organization. Employees must be trained to understand and trust the new technology, and stakeholders must be engaged to ensure successful adoption. Change management strategies should be implemented to address potential resistance and facilitate a smooth transition.
The Future of Machine Learning in Demand Forecasting
As machine learning technology continues to evolve, its applications in demand forecasting are expected to expand. Emerging trends such as real-time data processing, advanced analytics, and artificial intelligence are set to further enhance the accuracy and efficiency of demand forecasts. Businesses that embrace these innovations will be well-positioned to thrive in an increasingly competitive marketplace.
In conclusion, machine learning is revolutionizing the field of demand forecasting by providing businesses with more accurate, timely, and actionable insights. By leveraging this powerful technology, companies can optimize their operations, reduce costs, and better meet the needs of their customers. As the demand for machine learning in demand forecasting grows, businesses that invest in this technology will gain a significant competitive advantage.