Revolutionizing Fashion: The Impact of AI on Apparel Demand Forecasting
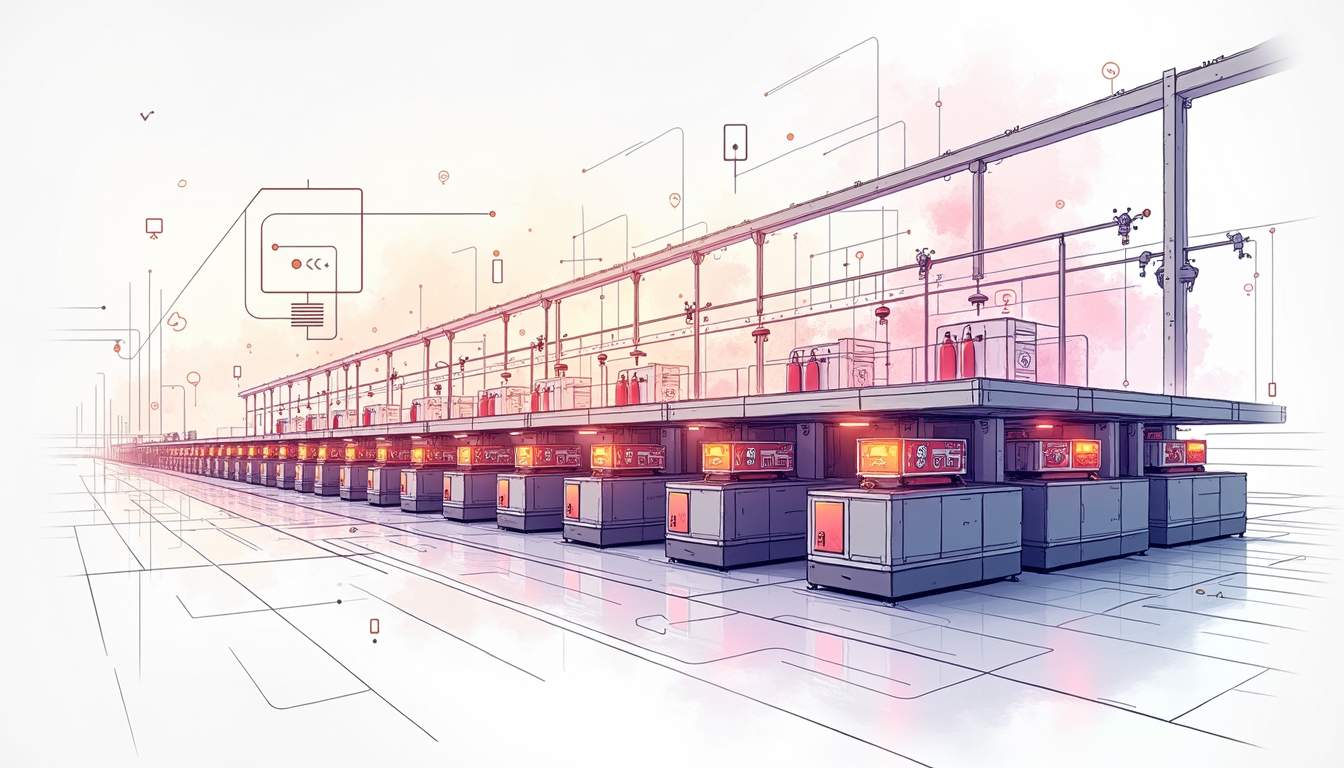
The fashion industry is undergoing a transformative journey, largely driven by advancements in artificial intelligence (AI). Among these advancements, one area that stands out is demand forecasting. By leveraging AI technology, apparel brands are refining their understanding of consumer behaviors and trends. This article delves into the crucial aspects of demand forecasting in fashion and how AI is revolutionizing this essential component of business strategy.
Understanding Demand Forecasting in the Fashion Sector
Demand forecasting plays a pivotal role in the fashion sector, helping brands anticipate future product demand based on historical sales data and market trends. The objective is simple yet profound: to ensure that the right products are available at the right time in the right quantities. Historically, fashion brands relied on intuition and periodic market research to make these predictions, but the landscape is changing.
The introduction of AI in this field has allowed brands to analyze vast datasets in real-time, incorporating variables such as weather patterns, social media trends, and even economic indicators, which heavily influence consumer behavior. Through advanced algorithms, brands can now develop more accurate predictions that help them stay ahead of the curve.
This shift from traditional methods to AI-driven insights signals a new era in fashion forecasting, where data becomes not just supportive but foundational to strategies applied at all levels of the business. For instance, brands can now leverage machine learning to identify emerging trends before they become mainstream, allowing them to capitalize on consumer interests as they evolve. This proactive approach not only enhances inventory management but also minimizes the risk of overproduction, a critical factor in an industry often criticized for its environmental impact.
Moreover, the integration of demand forecasting with supply chain management has led to more agile operations. Retailers can optimize their production schedules and distribution strategies based on real-time insights, ensuring that they respond swiftly to shifts in consumer preferences. This agility is particularly crucial during peak shopping seasons or unexpected market disruptions, where timely decision-making can significantly affect a brand's bottom line. As fashion continues to embrace sustainability, accurate demand forecasting will also play a vital role in reducing waste and promoting ethical consumption practices, aligning business objectives with consumer values.
Advantages of Demand Forecasting in Apparel
The benefits of effective demand forecasting in the apparel industry are manifold. As brands deploy AI-driven forecasting models, they unlock several advantages that can markedly improve their operational efficiency and customer experience.
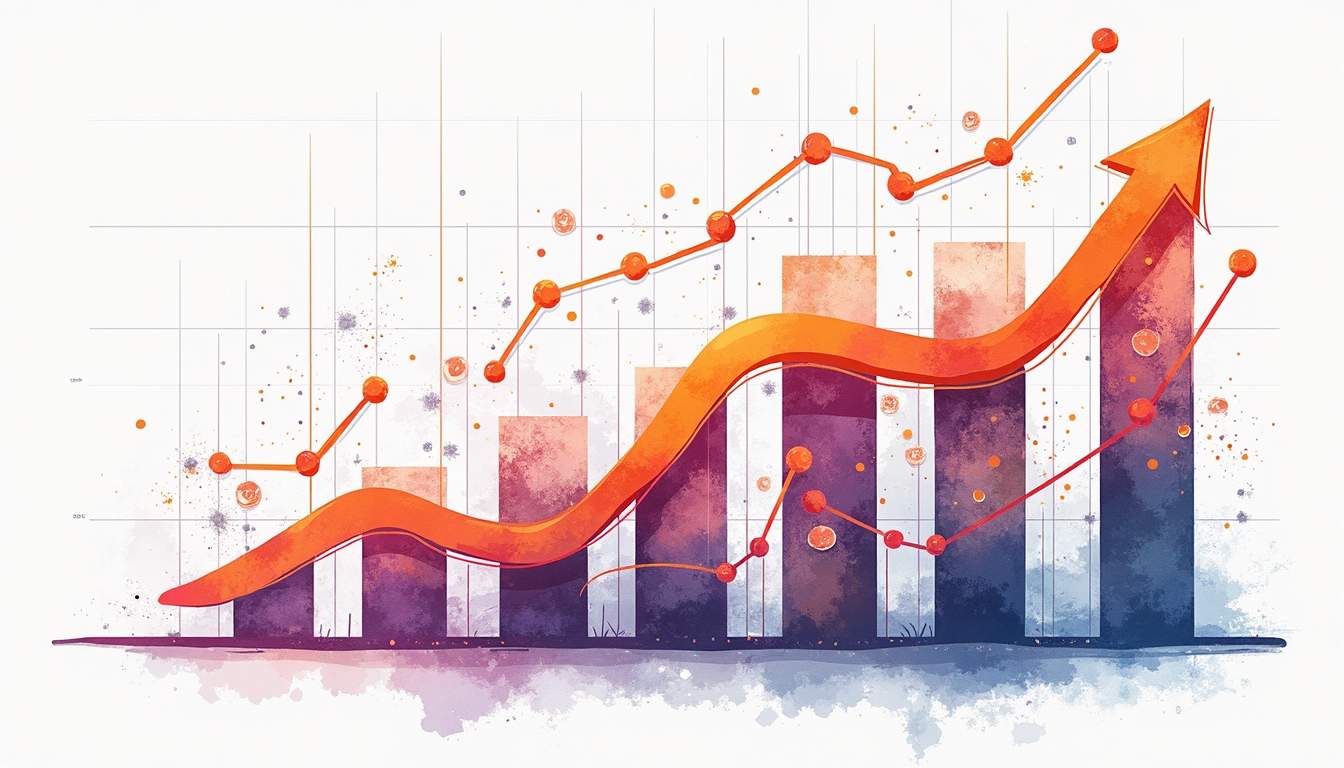
1. Real-Time Insights from Historical Trends
AI-powered demand forecasting takes historical data and delivers insights in real-time. This means that brands can react swiftly to changing market conditions instead of waiting for quarterly reports. Leveraging machine learning algorithms, businesses can dig deep into past trends and paint a clearer picture of future demand patterns.
For example, a sudden spike in demand for a particular style or color can be identified and addressed almost immediately, allowing brands to adjust production schedules and marketing efforts proactively. Such agility ensures they remain competitive, even in volatile markets. Additionally, real-time insights can help brands identify emerging trends before they become mainstream, giving them a first-mover advantage in the fast-paced fashion landscape.
2. Optimizing Inventory Control
Effective demand forecasting is critical for optimizing inventory levels. By employing AI, brands can predict which items will be popular and stock accordingly, minimizing overproduction and waste. This not only saves costs but also enhances sustainability practices.
With improved inventory control, businesses can achieve a balanced supply chain. This balance significantly reduces the burden of excess inventory, which can lead to markdowns and financial losses. Additionally, optimized inventory supports better cash flow, enabling brands to reinvest in innovation. The ability to maintain optimal stock levels also allows brands to respond to seasonal fluctuations more effectively, ensuring that they are well-prepared for peak shopping periods without overcommitting resources.
3. Boosting Customer Satisfaction
Customer satisfaction is fundamentally linked to availability. When brands can accurately predict what products will be in demand, they increase their chances of having those items on hand. This leads to happier customers; they find what they need when they shop, enhancing their overall experience.
Moreover, personalized shopping experiences are enriched through demand forecasting. By analyzing consumer interactions and preferences, brands can tailor their offerings to meet specific customer needs, solidifying brand loyalty in the process. Furthermore, by anticipating customer preferences, brands can create targeted marketing campaigns that resonate with their audience, ensuring that promotions are timely and relevant, which can further drive sales and enhance customer engagement.
4. Enhancing Efficiency in Time and Costs
AI-driven demand forecasting streamlines decision-making processes, allowing teams to focus on creative and strategic initiatives rather than routine data analysis. By automating forecasting processes, brands save precious time, which can be redirected toward product development, marketing strategies, and enhancing customer engagement.
Furthermore, this operational efficiency translates directly into cost savings. With accurate forecasts reducing the risks associated with excess inventory and stockouts, brands can enjoy improved profit margins and a more resilient business model. The integration of demand forecasting with other operational systems, such as supply chain management and sales tracking, can create a cohesive workflow that enhances overall business agility. This interconnectedness allows for quicker adjustments in strategy, ensuring that brands remain responsive to both market demands and consumer trends.
Obstacles in Demand Forecasting
Despite the substantial benefits that AI offers for demand forecasting, various challenges persist in this rapidly evolving field. Brands must navigate these obstacles carefully to harness the full potential of AI in their forecasting efforts.
1. Reliance on Up-to-Date Data
AI systems thrive on data, but the quality of that data is paramount. Brands must consistently feed their models with up-to-date information to ensure accurate forecasting results. Outdated or incomplete datasets can lead to erroneous predictions, hampering the overall effectiveness of an organization’s forecasting efforts.
Thus, establishing efficient data collection methods and encouraging a culture of data accuracy are essential components of a successful AI-driven forecasting initiative. This involves not only leveraging advanced technologies for data gathering but also implementing rigorous data validation processes. Regular audits of data sources can help in identifying discrepancies and ensuring that the information fed into AI systems reflects the most current market conditions and consumer behaviors.
2. Complexity and Resource Demands
Implementing AI solutions for demand forecasting is not without its complexities. The integration of advanced technologies requires significant investment in resources—ranging from personnel trained in data science to sophisticated software tools. Many brands, particularly smaller ones, can find the resource demands daunting.
Moreover, as technologies continue to evolve, ongoing education and adaptation are crucial. Companies must foster a culture of continuous learning to stay abreast of the latest developments in demand forecasting. This can include partnerships with educational institutions or participation in industry workshops that focus on emerging AI trends. By investing in their workforce, brands can not only enhance their forecasting capabilities but also build a more resilient organization that can adapt to future challenges in the market.
3. Managing Returns and Exchanges
Returns and exchanges remain a significant concern for fashion retailers. Poorly predicted demand can lead to excessive returns, straining logistics and financial performance. While AI can improve forecasting accuracy, it cannot eliminate the inherent unpredictability of consumer behavior.
Brands must develop robust return management strategies that work in tandem with demand forecasting practices, striking a balance between accommodating customer satisfaction and minimizing operational burdens. This could involve implementing more flexible return policies that adapt based on predictive analytics, as well as utilizing AI to analyze return patterns and identify root causes. By understanding why products are returned, brands can refine their forecasting models and improve inventory management, ultimately enhancing the customer experience while reducing unnecessary costs.
4. Challenges of Multichannel Forecasting
As consumers increasingly shop across various channels—from brick-and-mortar stores to online marketplaces—multichannel forecasting becomes even more complex. Different channels may exhibit varying trends and consumer preferences, making it essential for brands to unify their forecasting initiatives across all platforms.
To effectively harness AI in this capacity, organizations must invest in integrated systems capable of pulling data from multiple sources, ensuring a holistic view of consumer demand. This may also involve the use of advanced analytics to identify cross-channel influences, such as how online promotions affect in-store sales. Furthermore, brands should consider employing machine learning algorithms that can adapt to changing consumer behaviors in real-time, allowing for more agile forecasting that can respond to market dynamics as they unfold. By embracing a comprehensive multichannel strategy, brands can enhance their responsiveness to consumer needs and optimize their inventory across all touchpoints.
Demand Forecasting Solutions for the Fashion Industry
The complexities of demand forecasting in fashion have led to a rise in innovative solutions tailored specifically for the industry. With various tools at their disposal, fashion brands can leverage cutting-edge technology to enhance their forecasting accuracy and streamline operations.

1. Effortless Integration with E-commerce Platforms
A growing number of demand forecasting solutions offer seamless integration with existing e-commerce platforms. By creating a connected ecosystem, brands can gather real-time sales data and customer behavior insights across all points of sale. This information is pivotal for developing accurate forecasts.
Such integration enhances the automatic flow of information, enabling brands to make quick and informed decisions in response to shifting consumer preferences and trends. Moreover, the ability to track customer interactions and purchasing patterns allows brands to tailor their marketing strategies, ensuring that promotions resonate with their target audience. This level of personalization not only boosts sales but also fosters customer loyalty, as consumers feel understood and valued by the brands they support.
2. Valuable Insights for Operational Teams
AI-driven forecasting solutions not only assist in predicting demand but also provide invaluable insights for operational teams. By correlating data with production, procurement, and logistics, brands can optimize their entire supply chain process.
This collaboration ensures that everyone within the organization—from marketing to inventory management—is aligned with the same data-driven vision, culminating in a more unified approach to business strategy. Additionally, these insights can help identify potential bottlenecks in the supply chain, allowing for proactive adjustments that minimize delays and reduce costs. With a clearer understanding of inventory turnover rates and seasonal demand fluctuations, brands can maintain optimal stock levels, reducing the risk of overproduction or stockouts that could lead to lost sales.
3. Strategic Revenue and Sales Forecasting
By employing sophisticated AI algorithms, apparel brands can achieve strategic revenue and sales forecasting that extends beyond mere trend prediction. These forecasts can inform pricing strategies, promotional campaigns, and inventory management for various product lines.
The use of advanced analytics allows brands to simulate different market scenarios, helping them to devise proactive strategies that can mitigate risk and capitalize on opportunities when they arise. Furthermore, the ability to analyze historical data alongside current market trends enables brands to anticipate shifts in consumer behavior, such as the increasing demand for sustainable fashion. This foresight empowers brands to adjust their offerings and marketing messages accordingly, ensuring they remain relevant and competitive in an ever-evolving landscape.
In addition, leveraging data analytics can aid in identifying emerging markets and demographics that may have previously been overlooked. By understanding these new customer segments, brands can tailor their collections and marketing efforts to meet the unique preferences and needs of these audiences, ultimately driving growth and expanding their market reach.